With urban flood risk escalating because of climate change and rapid urbanization there is a pressing need for fast, accurate, high-resolution spatiotemporal nowcasting flood simulations. Although deep learning (DL) methods hold great promise for providing such information, they nevertheless confront two main challenges: how to capture multi-scale nonlinear spatiotemporal interdependencies, and how to optimize long-sequence generalization with limited computational resources.
Professor Huapeng Qin’s lab at the School of Environment and Energy, Peking University Shenzhen Graduate School, proposes a novel latent autoregressive U-like Recurrent Neural Network (U-RNN) to model the spatiotemporal dynamics of urban flooding. In addition, they introduce a Sliding Window-based Pre-warming (SWP) training paradigm that significantly reduces computational demands while improving the generalization for full-sequence predictions. A large-scale rainfall–urban flooding dataset is also constructed to serve as a benchmark for model evaluation.
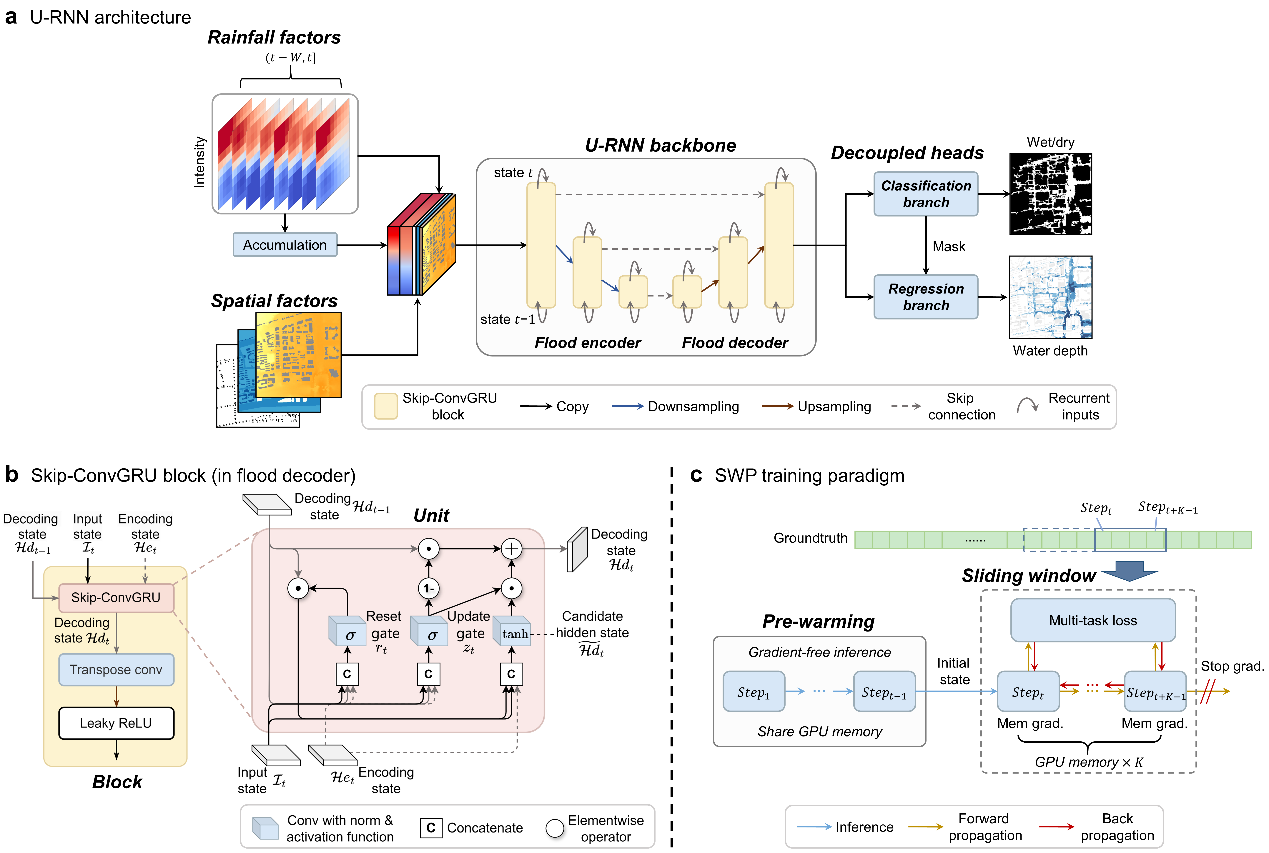
Figure 1. U-RNN for urban flood nowcasting. a, Architecture of U-RNN, a U-like recurrent neural network for urban flood spatiotemporal nowcasting. The flood encoder receives external factors (including rainfall time-series factors and spatial factors) and previous internal encoding states of urban flood systems, and then extracts multi-scale encoding states by Skip-ConvGRU blocks. These states are passed to the flood decoder via skip connections and transformed into decoding states via the Skip-ConvGRU blocks. These are then decoupled by classification and regression branches to nowcast flood inundation extent and water depth. b, Example of Skip-ConvGRU block used as flood decoder. It jointly extracts spatiotemporal features across layers by fusing the previous decoding state, previous block output, and the corresponding scale encoding state into Skip-ConvGRU. The unit, including a reset gate, an update gate and a candidate hidden state, processes the states in a tensor structure by means of convolution operators to jointly extract spatiotemporal features across the neural network layers. c, SWP training paradigm. It uses a sliding window of length K for gradient-based parameter optimization in each training iteration, storing only K times forward propagation and backpropagation parameter gradients into GPU memory. Window initial states are provided by gradient-free inference that involves sharing of the GPU memory, with later sequences after the window excluded from a given iteration. The base map in (a) is from OpenStreetMap, released under the Creative Commons CC BY-SA 2.0 license.
Case studies of four highly urbanized catchments in China and the United Kingdom, subjected to spatially uniform or variable rainfall events with return periods ranging from 0.1 to 500 years, demonstrate that U-RNN achieves high predictive accuracy in terms of flood extent, peak depth, and hydrograph, and accelerates 6-hour ahead nowcasts by more than 100 times when benchmarked against a state-of-the-art hydrodynamic model. Our method also significantly outperforms the state-of-the-art DL method at high spatiotemporal resolution of order O(m) in space and O(min) in time.
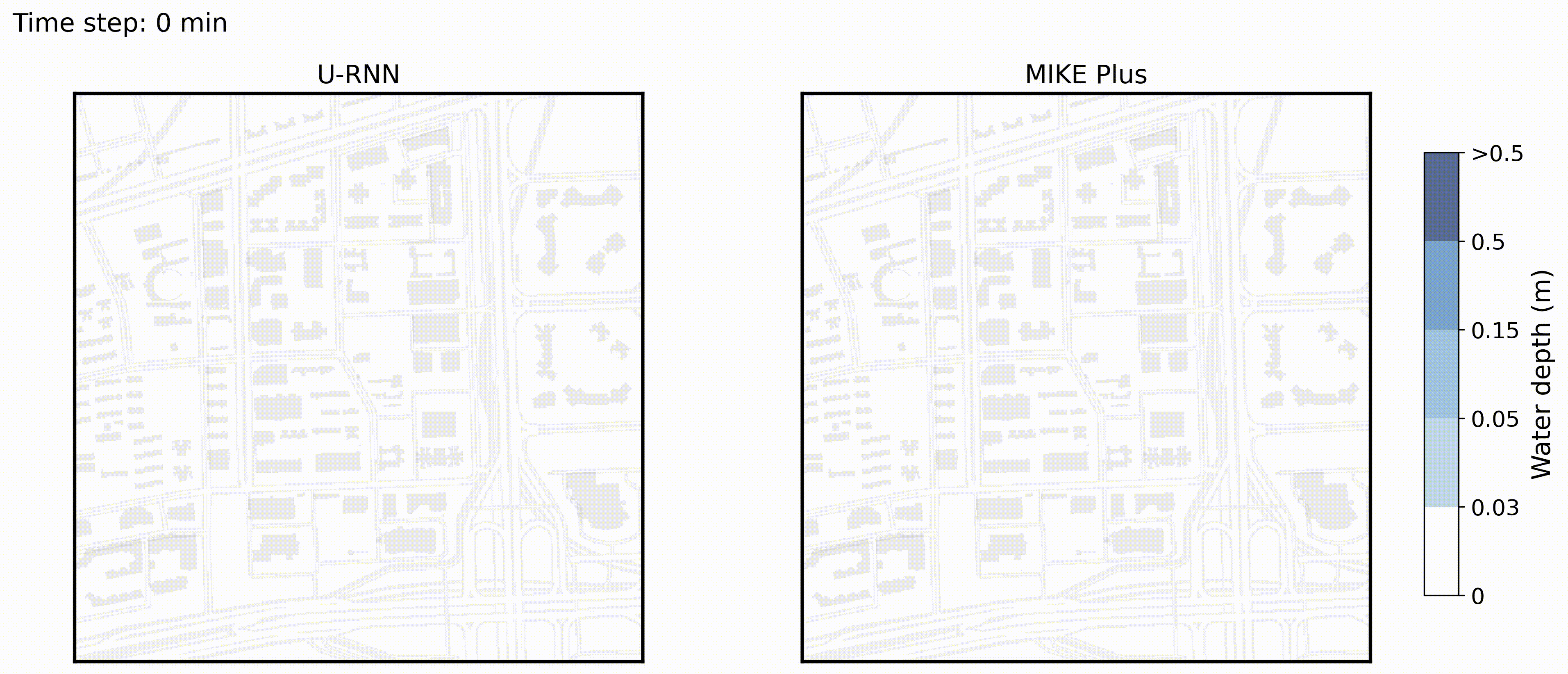
Figure 2. Comparison of urban flood spatiotemporal nowcasting between U-RNN and the commercial software MIKE+.
Author Biographies
The research work titled "U-RNN: High-resolution Spatiotemporal Nowcasting of Urban Flooding" has been published in the Journal of Hydrology. The first author of the paper is Dr. Xiaoyan Cao, a Ph.D. candidate at Peking University Shenzhen Graduate School, and the corresponding author is Prof. Huapeng Qin. Other key contributors include Baoying Wang, a Ph.D. student at Peking University Shenzhen Graduate School, who was responsible for dataset construction, and Dr. Yao Yao from Tsinghua University, who contributed to algorithm development. The research also benefited from guidance by Prof. Guangtao Fu of the University of Exeter and Prof. Alistair G.L. Borthwick of the University of Edinburgh, a Fellow of the Royal Academy of Engineering (UK). The project was supported by the Shenzhen Science and Technology Planning Program and the Innovation Team Project for General Universities in Guangdong Province. High-performance computing resources were provided by the School of Environment and Energy, Peking University Shenzhen Graduate School.